What Is a Good Relationship Based on
The relationship between evidence-based and data-driven medicine
The potential biases of evidence-based medicine can be overcome with data-driven medicine, now that we are amassing large data sets from which AI algorithms can learn and discover.
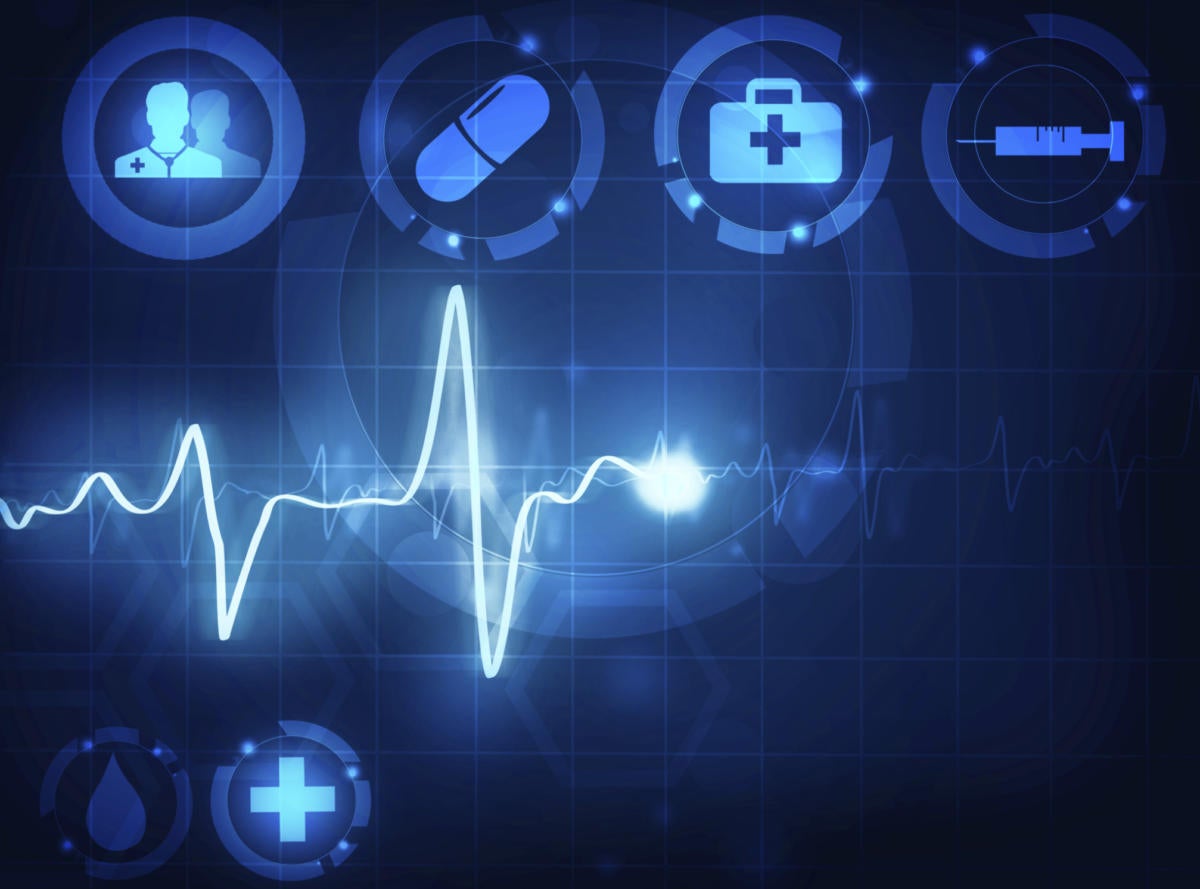
The term "artificial intelligence (AI) in healthcare" has become the current hot topic in health IT circles. Like with previous hot topics ("big data in healthcare," "interoperability," "patient engagement"), AI in healthcare will become a standard, basic element of every product on the market. Of course, the term means different things to different people, so when AI in healthcare is discussed in forums, or on blogs online, there are many different things that are really being referenced. At Flow Health, we have looked into how to apply insights from medical and genomic knowledge for precision medicine, and have examined how close we are to using AI for automated assistance in healthcare.
Some element of machine learning and automated assistance will become a routine part of every successful healthcare enterprise – from documentation and diagnosis assistance, to individualized medication suggestions, to plain-language explanations of personalized medical questions for consumers/patients, to treatment suggestions (such as for cancer care), to imaging interpretation assistance. All these activities will involve some element of AI as this technology matures.
A more fundamental issue arises as AI in healthcare becomes more robust, learns patterns from larger data sets, and uncovers insights previously hidden. That issue is this: what is the difference between evidence-based medicine (the gold standard for care, though frustratingly incompletely followed) and data-driven medicine? This topic is worth a closer look.
Evidence-based medicine
"Evidence-based medicine" represents the current best-practices foundation to the practice of modern medicine. It is the conscientious, explicit and judicious use of current best evidence in making decisions about the care of individual patients. It is based on observations that come from clinical studies of populations. The gold standard is the double-blind prospective study, where an adequate sample of patients is selected, some are given a particular treatment and some others are given either a different treatment or a placebo (and no one -- neither the patients nor the researchers -- knows who gets what), and then a measurable endpoint is analyzed, based on the response.
This is the basis of a scientific approach in medicine. A hypothesis is created (a clinical question is asked), and then tested with an experiment; the result either confirms or argues against the original hypothesis. A hypothesis can be something like this: patients with severe asthma (defined by a trip to the emergency department for asthma-related symptoms during the previous year) should do better with inhaled corticosteroids. The test would then be to select a sampling of such patients, given them all inhalers (some of them corticosteroids and some of them placebos), and observe the outcome (measured by number of trips to the emergency department over the ensuing time interval, say a year). The results would thus either support or argue against the original hypothesis.
Clearly, this is a more rational basis for making treatment recommendations than the "this could work - let's try this and see what happens" unscientific approach, based on random anecdotes. It is the backbone of modern medicine. The subtler problem here is that a human must postulate a hypothesis in order to initiate a study. This opens a door to cognitive bias. The questions that are asked, and the tests that are carried out are subject to prejudice, such as selective funding from money sources – for example, a pharmaceutical company that wants to fund a study showing their new product is better than placebo, but not looking at whether it is better than other treatments already on the market, or from competitors; or a government ban on funding studies that ask certain kinds of questions. It is the fundamental human bias in the hypothesis-creating (and subsequent testing and conclusions) that remains a weak spot in "evidence-based medicine."
Data-driven medicine
As AI matures, and patterns in very large data sets are discovered, we can now see the emergence of the next step beyond the hypothesis-testing-conclusions paradigm (which is the best we have now), and its attendant susceptibility to bias.
A machine-learning algorithm simply looks at data that already exists, and its precision and usefulness is a function of the data it has learned from. It does not have a particular bias. It discovers patterns that are found in already-existing data to form the basis for predictions. Machine learning is based on observing the relationship between different kinds of data objects. When the data objects are medical elements, and vast amounts of data are analyzed, associations emerge that are simply descriptive of experience found in the data. There are two kinds of pattern-search that one can think of: survey and drill-down. A survey algorithm[1] [2] simply looks for signal-to-noise blips in the data stream, without preconceived suppositions. If there is an interesting blip, then a drill-down can be done to look at a specific question (e.g., is there an association between the use of proton-pump inhibitors such as omeprazole and heart disease?).
The goal of data-driven medicine is to use retrospective data analysis to make better decisions, and inform predictions in a real-time way. Data can be used to assess all the variables that pertain to a specific individual and make precise, personalized recommendations that could not come from population-based studies.[3] [4] A type of question that can be answered by a data-driven approach that would be very difficult with the traditional evidenced-based approach might be something like this: for this specific patient, with these genetic markers, these labs, and this history – will she be the rare individual who will develop Achilles tendon inflammation and even rupture when given levofloxacin for a kidney infection? Can I know at the point of care, when considering writing a prescription?
It may well be that prospective studies will be a way of testing new treatment options (medications, other interventions) where there is no existing data yet, but the day-to-day practice of medicine will be increasingly data-driven.
Conclusions
The backbone of clinical medicine has been "evidence-based medicine," relying on studies done prospectively, over long study intervals, yet subject to a variety of biases. These potential biases can be overcome with data-driven medicine, now that we are amassing large data sets from which AI algorithms can learn and discover associations between medical data elements that might not have been previously seen. Data-driven medicine, while free from the cognitive biases inherent in humans postulating hypotheses, is retrospective, observing patterns in data already in existence. It will become the precision, real-time approach used in day-to-day medicine. This represents a true paradigm shift in how we look at medical discovery, and may emerge as the new engine driving individualized, personalized medicine of the future.
Dr. Robert Rowley is a practicing family medicine physician, health IT consultant and entrepreneur and active blogger. With Flow Health, he is particularly interested in bringing artificial intelligence and a medical knowledge graph to the field of medicine.
Copyright © 2017 IDG Communications, Inc.
What Is a Good Relationship Based on
Source: https://www.cio.com/article/3235025/the-relationship-between-evidence-based-and-data-driven-medicine.html
0 Response to "What Is a Good Relationship Based on"
Post a Comment